Determination of reactivity and neutron flux using modified neural network for HTGR
改良版ニューラルネットワーク・モデルを用いた高温ガス炉の反応度および中性子束の評価
Subekti, M.*; 工藤 和彦*; 鍋島 邦彦
; 高松 邦吉
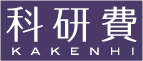
Subekti, M.*; Kudo, Kazuhiko*; Nabeshima, Kunihiko; Takamatsu, Kuniyoshi
HTTR炉心の中心制御棒を引き抜く反応度添加抜試験を評価する際、1点炉近似の動特性モデルを用いた解析手法は、最も汎用的である。一方、制御棒が引き抜かれると同時に、将来の反応度および中性子束の変化予測値を速やかに出力するには、非常に速い処理速度を持つ別の解析手法を必要とする。そこで、Time Delayed Neural Network (TDNN)とJordan Recurrent Neural Network (JordanRNN)を組み合わせ、新たにTD-Jordan RNNというニューラルネットワーク・モデルを作成し、HTTRの試験データをオフラインで十分学習させた。その結果、反応度添加試験時の反応度および中性子束の変化予測値を速やかに出力することができた。
Reactor kinetics based on point kinetic model have been generally applied as the standard method for neutronics codes. As the central control rod (C-CR) withdrawal test has demonstrated in a prismatic core of HTTR, the transient calculation of kinetic parameter, such as reactivity and neutron fluxes, requires a new method to shorten calculation-process time. Development of neural network method was applied to point kinetic model as the necessity of real-time calculation that could work in parallel with the digital reactivity meter. The combination of TDNN and Jordan RNN, such as TD-Jordan RNN, was the result of the modeling approach. The application of TD-Jordan RNN with adequate learning, tested offline, determined results accurately even when signal inputs were noisy. Furthermore, the preprocessing for neural network input utilized noise reduction as one of the equations to transform two of twelve time-delayed inputs into power corrected inputs.