Nuclear data generation using machine learning
機械学習を用いた核データの生成
岩元 大樹
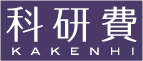
Iwamoto, Hiroki
発表者は、ガウス過程回帰を用いて、核データを生成する手法を開発した[1]。本手法は機械学習における教師あり学習法の一つであり、測定データを訓練データとみなして核データを生成する。カーネル関数および超パラメータの設定のみで、ノンパラメトリックな手法を用いて回帰を行うことができ、過学習の影響を受けにくいといった特長を有する。さらに、ベイズ推論を基にしているため、任意のエネルギー点における断面積の情報を不確実性を含めて生成することができる。本研究会では、ガウス過程の基礎について講演するとともに、本手法を用いた最新の研究成果および現在進行中の研究内容について報告する。[1] H. Iwamoto, "Generation of nuclear data using Gaussian process regression", Journal of Nuclear Science and Technology, 50:8, 932-938 (2020).
We have developed a method to generate nuclear data using Gaussian process regression [1], which is one of the machine learning technique. This method generates nuclear data by treating measured data as the training data in machine learning. Since Gaussian process regression is based on nonparametric Bayesian inference, the generated nuclear data are expressed as a predictive distribution including uncertainty information. In this presentation, the basics of the Gaussian process model, some examples of the application to nuclear data generation, and other related topics will be presented. [1] H. Iwamoto, "Generation of nuclear data using Gaussian process regression", Journal of Nuclear Science and Technology, 50:8, 932-938 (2020).