超音波フェーズドアレイ画像の機械学習による傷探傷
Machine learning of ultrasonic phased-array images for flaw detection
富田 直樹*; 古谷 正祐*; 朝日 学*; 久持 陸也*; 豊田 晃大
; 矢田 浩基 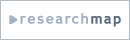
Tomita, Naoki*; Furuya, Masahiro*; Asahi, Manabu*; Hisamochi, Rikuya*; Toyota, Kodai; Yada, Hiroki
超音波フェーズドアレイはレーダー分野で開発された位相合成画像技術であり、原子力発電所における供用期間中検査等にも用いられている。ISIにおける超音波探傷試験(UT)は、一般的に配管や容器などの溶接部に対して外表面から板厚内の亀裂を検知するために行われるが、複雑形状や探傷位置から離れた部位に対する検査のニーズも存在する。本研究では、複雑形状に対する探傷精度向上を目的に、まず、フェーズドアレイUTを用いた複雑形状の試験片の探傷試験を行った。次に、得られた探傷画像を基に、19種類の事前学習ネットワークを使用した転移学習を行い、きずに対して高い判別精度が得られることを示した。
Ultrasonic phased array is a phase composite imaging technology developed in the radar field and has recently been used for nondestructive inspection of power generation equipment. However, scattered waves in the inspection target make it difficult to distinguish a flaw from scattering from the edge surface. In this study, we developed a method to discriminate flaws with high accuracy by adjusting parameters such as output voltage and receiver sensitivity to make it easier to see the flaws and by deep learning of the optimized flaw images. First, actual measurements were made using an ultrasonic phased-array flaw detector on a stainless-steel specimen. Next, a model was created to discriminate the presence or absence of flaws using transition learning, one of the machine learning methods. As a result, we found that the highest accuracy was achieved when transition learning was performed using inceptionv3 and resnet101, a convolutional neural network architecture. These results show that the method developed in this study is effective for nondestructive inspection.