An Attention-based anomaly detection model for ensuring safety in Nuclear Power Plants
Dong, F.*; Chen, S.*; Demachi, Kazuyuki*; Yoshikawa, Masanori
; Seki, Akiyuki
; Takaya, Shigeru
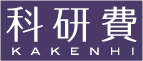
Timely and accurate anomaly detection for ensuring nuclear safety is at the top priority in the maintenance and management of NPPs (Nuclear Power Plants), since any slight anomaly in the plants might lead to irreversible severe accidents and high economic costs. Currently, due to the remarkable performance, deep learning algorithms are widely used for anomaly detection. Nevertheless, anomalies in NPPs are difficult to define, sparsely occurring, and are accompanied by variable noise labels, which all increase the difficulty on anomaly detection tasks. Moreover, as for general deep learning models, when analyzing time series data, the unsolved problems such as the interpretation of causing reason and loss of temporal features also pose challenges for detection. To alleviate these issues, an attention-based anomaly detection model for ensuring safety in NPPs is proposed, constituted of Conv1D (one-dimension convolutional neural network) backbone and attention mechanism. The performance of the proposed model was experimentally evaluated on the on the HTGR (High Temperature Gas-cooled Reactor) anomaly cases dataset based on the analytical code ACCORD, which conducted anomalies independently across multiple instruments. At this stage, the input data were composed of 9 classes of anomalies. According to the experimental results, the effectiveness and feasibility of the proposed anomaly detection model on ensuring safety of NPPs are demonstrated.