Attention-based time series analysis for data-driven anomaly detection in nuclear power plants
Dong, F.*; Chen, S.*; 出町 和之*; 吉川 雅紀
; 関 暁之
; 高屋 茂
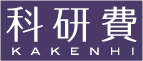
Dong, F.*; Chen, S.*; Demachi, Kazuyuki*; Yoshikawa, Masanori; Seki, Akiyuki; Takaya, Shigeru
To ensure nuclear safety, timely and accurate anomaly detection is of utmost importance in the daily condition monitoring of Nuclear Power Plants (NPPs), as any slight anomaly in a plant may result in an irreversible and serious accident, as well as high costs of maintenance and management. Nevertheless, due to the unique inherent attributes of anomalies, the difficulty of automatic detection in NPPs is increased. Previous model-driven anomaly detection approaches required skilled priori knowledge, leading to their limited usability. Commonly adopted deep learning-based data-driven anomaly detection approaches may not easily acquire the most relevant features when dealing with sensor data containing redundant information with uneven distribution of anomalies. To alleviate these issues, this paper propose an attention-based time series model for anomaly detection to ensure safety in NPPs. First, we employ one-dimension convolutional neural network (1D-CNN) backbone for feature extraction to preserve original inherent features of time series inputs. Subsequently, we originally adopt soft-attention mechanism to automatically extract the most relevant temporal features considering the specificity of anomaly detection in NPPs. The performance of the proposed model was experimentally validated on the High Temperature Gas-cooled Reactor (HTGR) anomaly case dataset simulated using the analytical code. The experimental results indicate that the proposed model was capable of detecting anomalies in NPPs with superior performance to the baseline model, while ensuring fast detection at short time steps.