レーザー溶断に伴う溶融物飛散挙動への機械学習の適用
Application of machine learning to spattering phenomena in laser cutting
楠本 利行*; 猿田 晃一
; 直江 崇
; 勅使河原 誠
; 二川 正敏
; 長谷川 和男*; 坪井 昭彦 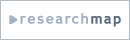
Kusumoto, Toshiyuki*; Saruta, Koichi; Naoe, Takashi; Teshigawara, Makoto; Futakawa, Masatoshi; Hasegawa, Kazuo*; Tsuboi, Akihiko
原子力施設の廃止措置や放射性廃棄物の減容化に、切断技術としてレーザー溶断を適用する場合、レーザーによる加熱で溶融した物質が、溶滴となって飛散するスパッタが発生するために、放射能汚染を生じる恐れがある。本研究では、レーザー溶断時のスパッタ飛散量の低減を目的に、レーザーパワー,ビーム径,照射時間からなるレーザー制御条件と高速度カメラで可視化したSS316L試験片のスパッタ飛散過程を3つの特徴的な挙動を示す時間領域:(I)金属蒸気の発生、(II)溶融金属の液膜的挙動と粒状化、(III)溶融金属の液柱化と断続的噴出に分類し評価した結果に対して機械学習を適用し、レーザー制御条件とスパッタ飛散挙動を関連付ける予測モデルを作成した。予測モデルの精度は89%であり、このモデルで計算したスパッタ飛散量の予測結果をレーザーパワーとビーム径の2次元ヒートマップとして図示することで、100点程度の少ない学習データでも予測結果の効率的な評価が可能となり、スパッタ飛散量を低減するレーザー制御条件の選定ができることを示した。
Reducing spatter, i.e., melt droplets flown out of the melt pool, is one of the critical issues when laser cutting is employed as a machining tool for radioactive wastes because the ejected droplets can lead to radioactive contamination with potential human exposure. The spattering phenomena are complicated processes that involve multiple physical phenomena, causing difficulty in the determination of laser parameters to minimize the amount of spatter. Here we observe the spatter ejected from 316L stainless steel plates using a high-speed camera and apply a machine learning technique to these captured images on the basis of three distinctive behaviors appeared at specific time intervals of the process of spattering phenomena: (I) a vapor, (II) a liquid film and breakup into droplets, and (III) a liquid capillary. The numerical model established through the machine learning technique predicts the spattering phenomena with an accuracy of 89% and can be used to determine the laser power and beam diameter that reduce the spatter eruption during laser irradiation.