A Numerical study on machine-learning-based ultrasound tomography of bubbly two-phase flows
Wada, Yuki
; Hirose, Yoshiyasu
; Shibamoto, Yasuteru 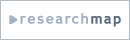
Ultrasound tomography of bubbly two-phase flows using machine learning (ML) was investigated by performing two-dimensional ultrasound numerical simulations using a finite element method simulator. To date, studies on ultrasound tomography for two-phase flow measurements have been conducted only for some bubbles. However, in an actual bubbly flow, numerous bubbles are complexly distributed in the cross-section of the flow channel. This limitation of previous studies originates from the transmission characteristics of ultrasound waves through a medium. The transmission characteristics of ultrasound waves are different from those of other probe signals, such as radiation, electrical, and optical signals. In this study, the feasibility of combining ultrasound tomography with ML was evaluated for dense bubble distributions with up to 20 bubbles (cross-sectional average void fraction of approximately 0.29). We investigated the effects of the temporal length of the received waveform and number of sensors to optimize the system on the prediction performance of the bubble distribution. The simultaneous driving of the installed sensors was simulated to reduce the measurement time for the entire cross-section and verify the applicability of the method. Thus, it was confirmed that ultrasound tomography using ML has sufficient prediction performance, even for a complex bubble distribution with many bubbles, and that the cross-sectional average void fraction can be predicted with high accuracy.