Atomic stress distribution near Al surfaces, calculated using artificial neural network interatomic potential
Lobzenko, I.
; Shiihara, Yoshinori*; Mori, Hideki*; Matsunaka, Daisuke*; Tsuru, Tomohito
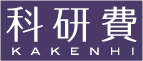
Recently methods of machine learning have become an important part of materials science. Particularly, interatomic potentials built using such methods demonstrate accuracy of geometrical characteristics of materials approaching the accuracy of first-principle calculations. In our work we use artificial neural networks to build potentials, and therefore they are called ANN potentials. We focus on the atomic stress, one of the important properties of materials, which can be calculated in classical approximation. Analysis of microscopic stress can be applied to any type of nonuniform system (such as defects in bulk, two-dimensional crystals, molecules assemblies, etc.). We show how the central-force decomposition (CFD) scheme can be used in the framework of ANN potentials for the derivation of atomic stress tensor. It is important to use CFD due to the fact that the symmetry of the stress tensor may be broken in other schemes. Finally, we calculate atomic stress distributions near surfaces of pure Al with different orientations. It is known from first-principle studies that there is a charge oscillations near Al surfaces, however it cannot be captured by existing interatomic potentials. Our results, obtained with the new ANN potential, show oscillations of atomic stress near Al surface. Even though our potential was fitted to only energies of Al structures (calculated in quantum-mechanics approximation), we attribute the atomic stress oscillations to the charge distribution of the real system. Charge oscillations are affecting total energies of structures, and therefore are implicitly included in the data set, which we use for building the potential.