機械学習を活用した腐食画像認識
Recognition of corrosion image using machine learning
五十嵐 誉廣
; 大谷 恭平
; 青山 高士
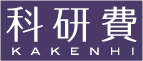
Igarashi, Takahiro; Otani, Kyohei; Aoyama, Takahito
橋梁やプラント等の大型構造物の健全性評価は重要課題の一つである。大型構造物の主要材料の一つである鉄鋼材料の腐食メカニズムを理解し材料健全性評価に応用するため、暴露試験や実験室における乾湿繰り返し試験等、多くの研究がなされている。また外観写真から腐食状態を予測する研究もなされており、破壊検査を行うことができない現物の腐食量を予測する手法として有用な方法の一つと考えられる。本報では、乾湿繰り返し試験を行った炭素鋼試験片の錆落とし前の外観写真に対してFAST特徴点抽出法を適用することにより、腐食深さの絶対値の予測は難しいが腐食位置については凡そ予測できることを示した。
Evaluation of the integrity of large structures such as bridges and plants is one of the most important issues. In order to understand the corrosion mechanisms of steels using these large structures and to apply them to the integrity evaluation, many studies have been conducted, including exposure tests and repeated wet and dry tests in the laboratory. In addition, another research approach has been conducted to predict the corrosion state from surface images, which is considered to be one of the most useful methods to predict the amount of corrosion of actual materials that cannot be destructively inspected. In this study, we show that the FAST feature point extraction method can be used to predict the location of corrosion, although it is difficult to predict the absolute value of corrosion depth, by applying the FAST feature point extraction method to surface images of carbon steel specimens that have been subjected to repeated wet and dry tests before rust removal.