Application of machine learning potentials in path integral molecular dynamics simulations
経路積分分子動力学シミュレーションにおける機械学習ポテンシャルの応用
Thomsen, B.
; 志賀 基之
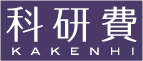
Thomsen, B.; Shiga, Motoyuki
量子力学のファインマン経路定式化に基づく経路積分分子動力学(PIMD)とその関連手法は、バルク相物質における核量子効果を直接モデリングする方法を提供する。しかし、これらの手法の各タイムステップでは、第一原理レベルで複数の電子構造エネルギー、勾配、応力ベクトルを評価する必要がある。そのため、第一原理レベルで追求するには、非常に計算コストがかかる。最近、機械学習ポテンシャル(MLP)が、PIMD法のコストを下げ、長時間のダイナミクスを第一原理精度で研究できる方法として提案されている。本発表では、パラジウム金属中の水素拡散を複数の温度にわたって研究した結果を紹介する。また、MLPを水とその同位体のPIMD研究に適用するための現在進行中の取り組みについても説明する。
Path integral molecular dynamics (PIMD) and related methods, based on the Feynman path formulation of quantum mechanics, offer a direct way of modelling nuclear quantum effects in bulk phase materials. Each timestep of these methods does however require the evaluation of several electronic structure energies, gradients and stress vectors at the ab initio level. They are thus very computationally expensive to pursue at the ab initio level. Recently machine learned potentials (MLPs) have been suggested as a way to bring down the cost and allow long time dynamics to be studied with ab initio accuracy for PIMD methods. In this presentation we present the results of studying hydrogen diffusion in Palladium metal across several temperatures. We will also discuss our ongoing efforts to apply MLPs to PIMD studies of water and its isotopologues.