機械学習を用いたJ-PARC RCS用ペイントバンプ電源の波形パターン制御
Waveform pattern control of paint bump power supply for J-PARC RCS using machine learning
杉田 萌
; 高柳 智弘
; 植野 智晶*; 小野 礼人; 堀野 光喜*; 金正 倫計
; 小栗 英知
; 山本 風海
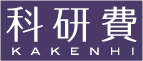
Sugita, Moe; Takayanagi, Tomohiro; Ueno, Tomoaki*; Ono, Ayato; Horino, Koki*; Kinsho, Michikazu; Oguri, Hidetomo; Yamamoto, Kazami
J-PARC RCSでは、ペイント入射のビーム軌道偏向にペイントバンプ電磁石を用いている。ペイントバンプ電磁石用の電源は、IGBT制御のチョッパ回路で構成され、指令電流と指令電圧のパターンにより、ビーム軌道を時間変化させる出力電流波形(ペイントパターン)を作成することができる。ビーム軌道の制御精度は、指令電流と出力電流の波形の形状差(出力電流偏差)で決まる。現在のペイントパターン調整では、電源制御の応答関数に応じてパターンを調整するソフトを用いたうえで手動調整を行い、要求精度を達成している。しかし、この調整に1時間程度を要しており、更なる調整時間の短縮を行いたい。また、より最適な調整方法によりビームロスの低減を実現するために、従来の要求より10倍精密な出力電流偏差を実現したい。高精度なペイントパターンの作成には、負荷側のインピーダンスの解析モデルが必要になるが、時間変化する非線形のペイントパターンにおいてはインピーダンスが変化するため、解析モデルの構築は非常に困難である。機械学習では、負荷の解析モデルを構築せずとも学習によって非線形な応答を高速かつ高精度で得ることが可能である。機械学習を用いて台形波の出力パターン調整を行ったところ、学習を重ねることで立ち上り直後のリンギングを抑制した出力パターンの作成に成功した。本発表ではシステムの現状と今後の展望について報告する。
In J-PARC RCS, paint bump magnets are used to displace the beam orbit during paint injection, which produces a high intensity beam. A pattern of command current and command voltage can be used to create an output current waveform that varies the beam orbit over time. The accuracy of beam orbit control is determined by the shape difference between the command current and output current waveforms. In the current paint pattern adjustment, a deviation of
1% or less is achieved by manual adjustment after using software that adjusts the pattern according to the response function of the power supply control. However, we would like to reduce the adjustment time. In addition, since the accuracy of paint injection is determined by the adjustment system of the paint magnet power supply, we would like to achieve output current deviation 10 times more precise than before to reduce beam loss. An analytical model of the load-side impedance is necessary to create a high-precision paint pattern, but it is very difficult to construct an analytical model because the load-side impedance changes in a time-varying nonlinear paint pattern. We used machine learning to adjust the output pattern of the paint pattern and achieved a deviation of less than
0.5% through repeated learning. This presentation will report on the current status of the system and its prospects.